Title |
Gradient Matching Methods for Computational Inference in Mechanistic Models for Systems Biology: A Review and Comparative Analysis
|
---|---|
Published in |
Frontiers in Bioengineering and Biotechnology, November 2015
|
DOI | 10.3389/fbioe.2015.00180 |
Pubmed ID | |
Authors |
Benn Macdonald, Dirk Husmeier |
Abstract |
Parameter inference in mathematical models of biological pathways, expressed as coupled ordinary differential equations (ODEs), is a challenging problem in contemporary systems biology. Conventional methods involve repeatedly solving the ODEs by numerical integration, which is computationally onerous and does not scale up to complex systems. Aimed at reducing the computational costs, new concepts based on gradient matching have recently been proposed in the computational statistics and machine learning literature. In a preliminary smoothing step, the time series data are interpolated; then, in a second step, the parameters of the ODEs are optimized, so as to minimize some metric measuring the difference between the slopes of the tangents to the interpolants, and the time derivatives from the ODEs. In this way, the ODEs never have to be solved explicitly. This review provides a concise methodological overview of the current state-of-the-art methods for gradient matching in ODEs, followed by an empirical comparative evaluation based on a set of widely used and representative benchmark data. |
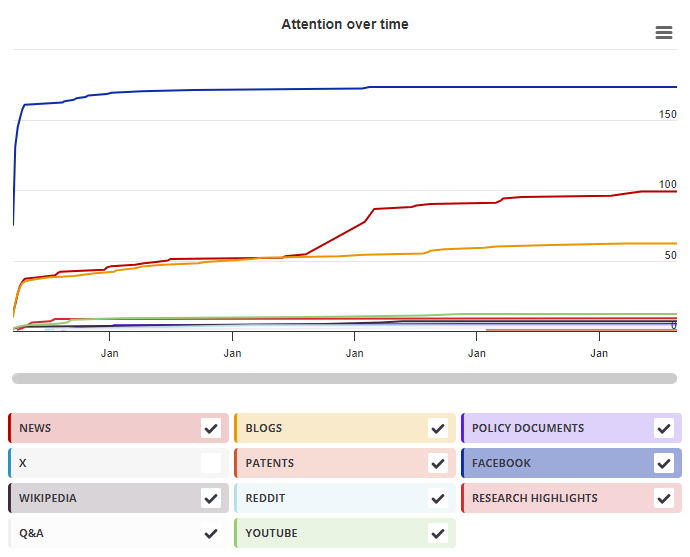
X Demographics
As of 1 July 2024, you may notice a temporary increase in the numbers of X profiles with Unknown location. Click here to learn more.
Geographical breakdown
Country | Count | As % |
---|---|---|
Switzerland | 1 | 100% |
Demographic breakdown
Type | Count | As % |
---|---|---|
Members of the public | 1 | 100% |
Mendeley readers
Geographical breakdown
Country | Count | As % |
---|---|---|
Switzerland | 1 | 2% |
Unknown | 53 | 98% |
Demographic breakdown
Readers by professional status | Count | As % |
---|---|---|
Student > Ph. D. Student | 18 | 33% |
Researcher | 11 | 20% |
Student > Master | 7 | 13% |
Student > Postgraduate | 6 | 11% |
Student > Doctoral Student | 1 | 2% |
Other | 4 | 7% |
Unknown | 7 | 13% |
Readers by discipline | Count | As % |
---|---|---|
Mathematics | 9 | 17% |
Engineering | 8 | 15% |
Agricultural and Biological Sciences | 7 | 13% |
Biochemistry, Genetics and Molecular Biology | 6 | 11% |
Computer Science | 5 | 9% |
Other | 7 | 13% |
Unknown | 12 | 22% |