Title |
A Bayesian Framework for the Classification of Microbial Gene Activity States
|
---|---|
Published in |
Frontiers in Microbiology, August 2016
|
DOI | 10.3389/fmicb.2016.01191 |
Pubmed ID | |
Authors |
Craig Disselkoen, Brian Greco, Kaitlyn Cook, Kristin Koch, Reginald Lerebours, Chase Viss, Joshua Cape, Elizabeth Held, Yonatan Ashenafi, Karen Fischer, Allyson Acosta, Mark Cunningham, Aaron A. Best, Matthew DeJongh, Nathan Tintle |
Abstract |
Numerous methods for classifying gene activity states based on gene expression data have been proposed for use in downstream applications, such as incorporating transcriptomics data into metabolic models in order to improve resulting flux predictions. These methods often attempt to classify gene activity for each gene in each experimental condition as belonging to one of two states: active (the gene product is part of an active cellular mechanism) or inactive (the cellular mechanism is not active). These existing methods of classifying gene activity states suffer from multiple limitations, including enforcing unrealistic constraints on the overall proportions of active and inactive genes, failing to leverage a priori knowledge of gene co-regulation, failing to account for differences between genes, and failing to provide statistically meaningful confidence estimates. We propose a flexible Bayesian approach to classifying gene activity states based on a Gaussian mixture model. The model integrates genome-wide transcriptomics data from multiple conditions and information about gene co-regulation to provide activity state confidence estimates for each gene in each condition. We compare the performance of our novel method to existing methods on both simulated data and real data from 907 E. coli gene expression arrays, as well as a comparison with experimentally measured flux values in 29 conditions, demonstrating that our method provides more consistent and accurate results than existing methods across a variety of metrics. |
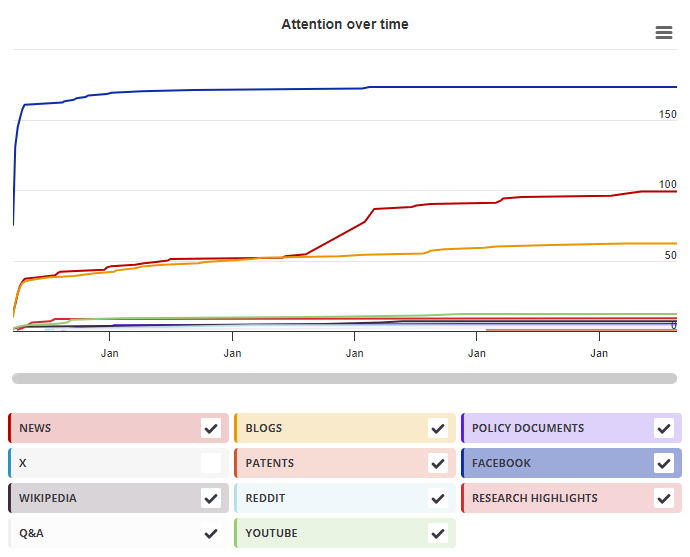
X Demographics
As of 1 July 2024, you may notice a temporary increase in the numbers of X profiles with Unknown location. Click here to learn more.
Geographical breakdown
Country | Count | As % |
---|---|---|
United States | 3 | 38% |
Chile | 1 | 13% |
Switzerland | 1 | 13% |
Unknown | 3 | 38% |
Demographic breakdown
Type | Count | As % |
---|---|---|
Scientists | 6 | 75% |
Members of the public | 2 | 25% |
Mendeley readers
Geographical breakdown
Country | Count | As % |
---|---|---|
Unknown | 11 | 100% |
Demographic breakdown
Readers by professional status | Count | As % |
---|---|---|
Student > Ph. D. Student | 3 | 27% |
Other | 2 | 18% |
Student > Master | 2 | 18% |
Professor > Associate Professor | 2 | 18% |
Researcher | 1 | 9% |
Other | 0 | 0% |
Unknown | 1 | 9% |
Readers by discipline | Count | As % |
---|---|---|
Agricultural and Biological Sciences | 6 | 55% |
Biochemistry, Genetics and Molecular Biology | 2 | 18% |
Environmental Science | 1 | 9% |
Unknown | 2 | 18% |