Title |
Feasibility Theory Reconciles and Informs Alternative Approaches to Neuromuscular Control
|
---|---|
Published in |
Frontiers in Computational Neuroscience, September 2018
|
DOI | 10.3389/fncom.2018.00062 |
Pubmed ID | |
Authors |
Brian A. Cohn, May Szedlák, Bernd Gärtner, Francisco J. Valero-Cuevas |
Abstract |
We present Feasibility Theory, a conceptual and computational framework to unify today's theories of neuromuscular control. We begin by describing how the musculoskeletal anatomy of the limb, the need to control individual tendons, and the physics of a motor task uniquely specify the family of all valid muscle activations that accomplish it (its 'feasible activation space'). For our example of producing static force with a finger driven by seven muscles, computational geometry characterizes-in a complete way-the structure of feasible activation spaces as 3-dimensional polytopes embedded in 7-D. The feasible activation space for a given task is the landscape where all neuromuscular learning, control, and performance must occur. This approach unifies current theories of neuromuscular control because the structure of feasible activation spaces can be separately approximated as either low-dimensional basis functions (synergies), high-dimensional joint probability distributions (Bayesian priors), or fitness landscapes (to optimize cost functions). |
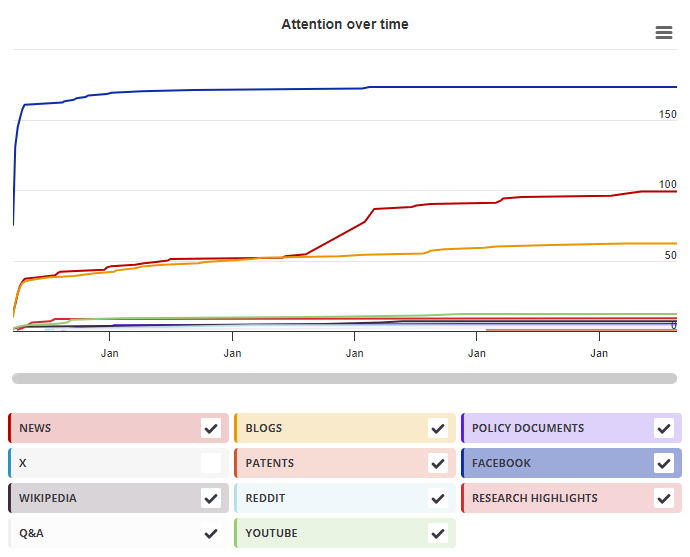
X Demographics
As of 1 July 2024, you may notice a temporary increase in the numbers of X profiles with Unknown location. Click here to learn more.
Geographical breakdown
Country | Count | As % |
---|---|---|
United States | 2 | 22% |
Switzerland | 1 | 11% |
Chile | 1 | 11% |
Unknown | 5 | 56% |
Demographic breakdown
Type | Count | As % |
---|---|---|
Members of the public | 9 | 100% |
Mendeley readers
Geographical breakdown
Country | Count | As % |
---|---|---|
Unknown | 62 | 100% |
Demographic breakdown
Readers by professional status | Count | As % |
---|---|---|
Student > Ph. D. Student | 18 | 29% |
Student > Bachelor | 7 | 11% |
Student > Master | 7 | 11% |
Researcher | 7 | 11% |
Student > Doctoral Student | 5 | 8% |
Other | 6 | 10% |
Unknown | 12 | 19% |
Readers by discipline | Count | As % |
---|---|---|
Engineering | 18 | 29% |
Sports and Recreations | 6 | 10% |
Nursing and Health Professions | 5 | 8% |
Neuroscience | 5 | 8% |
Agricultural and Biological Sciences | 3 | 5% |
Other | 8 | 13% |
Unknown | 17 | 27% |