Title |
Spike Detection for Large Neural Populations Using High Density Multielectrode Arrays
|
---|---|
Published in |
Frontiers in Neuroinformatics, December 2015
|
DOI | 10.3389/fninf.2015.00028 |
Pubmed ID | |
Authors |
Jens-Oliver Muthmann, Hayder Amin, Evelyne Sernagor, Alessandro Maccione, Dagmara Panas, Luca Berdondini, Upinder S. Bhalla, Matthias H. Hennig |
Abstract |
An emerging generation of high-density microelectrode arrays (MEAs) is now capable of recording spiking activity simultaneously from thousands of neurons with closely spaced electrodes. Reliable spike detection and analysis in such recordings is challenging due to the large amount of raw data and the dense sampling of spikes with closely spaced electrodes. Here, we present a highly efficient, online capable spike detection algorithm, and an offline method with improved detection rates, which enables estimation of spatial event locations at a resolution higher than that provided by the array by combining information from multiple electrodes. Data acquired with a 4096 channel MEA from neuronal cultures and the neonatal retina, as well as synthetic data, was used to test and validate these methods. We demonstrate that these algorithms outperform conventional methods due to a better noise estimate and an improved signal-to-noise ratio (SNR) through combining information from multiple electrodes. Finally, we present a new approach for analyzing population activity based on the characterization of the spatio-temporal event profile, which does not require the isolation of single units. Overall, we show how the improved spatial resolution provided by high density, large scale MEAs can be reliably exploited to characterize activity from large neural populations and brain circuits. |
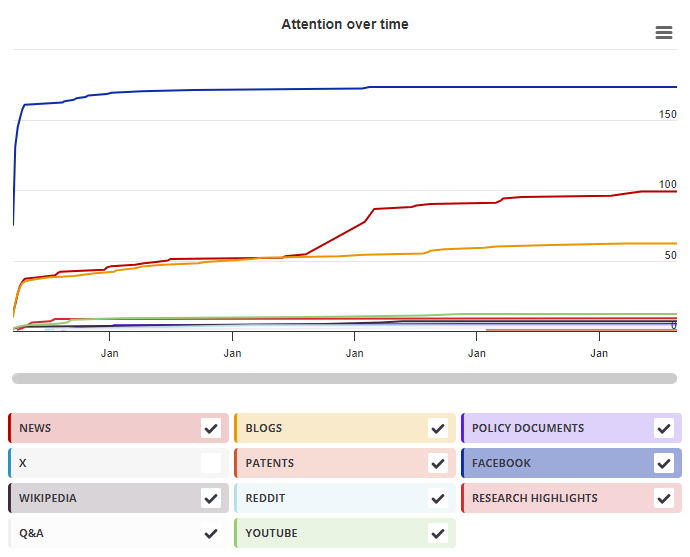
X Demographics
As of 1 July 2024, you may notice a temporary increase in the numbers of X profiles with Unknown location. Click here to learn more.
Geographical breakdown
Country | Count | As % |
---|---|---|
Germany | 1 | 33% |
United States | 1 | 33% |
Switzerland | 1 | 33% |
Demographic breakdown
Type | Count | As % |
---|---|---|
Members of the public | 2 | 67% |
Science communicators (journalists, bloggers, editors) | 1 | 33% |
Mendeley readers
Geographical breakdown
Country | Count | As % |
---|---|---|
United Kingdom | 4 | 3% |
Germany | 2 | 1% |
France | 1 | <1% |
Portugal | 1 | <1% |
Japan | 1 | <1% |
United States | 1 | <1% |
Unknown | 138 | 93% |
Demographic breakdown
Readers by professional status | Count | As % |
---|---|---|
Student > Ph. D. Student | 46 | 31% |
Researcher | 25 | 17% |
Student > Master | 18 | 12% |
Student > Bachelor | 10 | 7% |
Other | 7 | 5% |
Other | 17 | 11% |
Unknown | 25 | 17% |
Readers by discipline | Count | As % |
---|---|---|
Neuroscience | 41 | 28% |
Engineering | 28 | 19% |
Agricultural and Biological Sciences | 16 | 11% |
Computer Science | 10 | 7% |
Physics and Astronomy | 7 | 5% |
Other | 17 | 11% |
Unknown | 29 | 20% |