Title |
Pattern Recognition of Momentary Mental Workload Based on Multi-Channel Electrophysiological Data and Ensemble Convolutional Neural Networks
|
---|---|
Published in |
Frontiers in Neuroscience, May 2017
|
DOI | 10.3389/fnins.2017.00310 |
Pubmed ID | |
Authors |
Jianhua Zhang, Sunan Li, Rubin Wang |
Abstract |
In this paper, we deal with the Mental Workload (MWL) classification problem based on the measured physiological data. First we discussed the optimal depth (i.e., the number of hidden layers) and parameter optimization algorithms for the Convolutional Neural Networks (CNN). The base CNNs designed were tested according to five classification performance indices, namely Accuracy, Precision, F-measure, G-mean, and required training time. Then we developed an Ensemble Convolutional Neural Network (ECNN) to enhance the accuracy and robustness of the individual CNN model. For the ECNN design, three model aggregation approaches (weighted averaging, majority voting and stacking) were examined and a resampling strategy was used to enhance the diversity of individual CNN models. The results of MWL classification performance comparison indicated that the proposed ECNN framework can effectively improve MWL classification performance and is featured by entirely automatic feature extraction and MWL classification, when compared with traditional machine learning methods. |
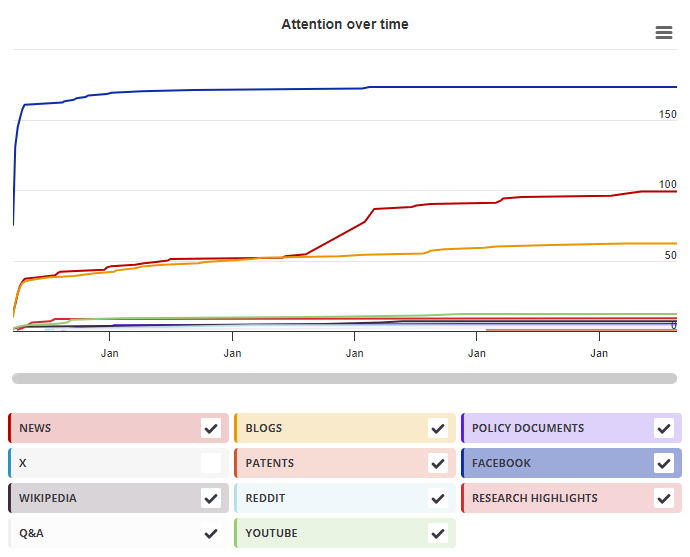
X Demographics
As of 1 July 2024, you may notice a temporary increase in the numbers of X profiles with Unknown location. Click here to learn more.
Geographical breakdown
Country | Count | As % |
---|---|---|
United States | 2 | 40% |
Switzerland | 1 | 20% |
Unknown | 2 | 40% |
Demographic breakdown
Type | Count | As % |
---|---|---|
Members of the public | 5 | 100% |
Mendeley readers
Geographical breakdown
Country | Count | As % |
---|---|---|
Unknown | 42 | 100% |
Demographic breakdown
Readers by professional status | Count | As % |
---|---|---|
Student > Ph. D. Student | 10 | 24% |
Student > Master | 8 | 19% |
Student > Doctoral Student | 5 | 12% |
Researcher | 3 | 7% |
Student > Bachelor | 2 | 5% |
Other | 4 | 10% |
Unknown | 10 | 24% |
Readers by discipline | Count | As % |
---|---|---|
Engineering | 11 | 26% |
Computer Science | 8 | 19% |
Medicine and Dentistry | 4 | 10% |
Psychology | 3 | 7% |
Neuroscience | 3 | 7% |
Other | 2 | 5% |
Unknown | 11 | 26% |