Title |
Grounding the Meanings in Sensorimotor Behavior using Reinforcement Learning
|
---|---|
Published in |
Frontiers in Neurorobotics, January 2012
|
DOI | 10.3389/fnbot.2012.00001 |
Pubmed ID | |
Authors |
Igor Farkaš, Tomáš Malík, Kristína Rebrová, Farkaš, Igor, Malík, Tomáš, Rebrová, Kristína |
Abstract |
The recent outburst of interest in cognitive developmental robotics is fueled by the ambition to propose ecologically plausible mechanisms of how, among other things, a learning agent/robot could ground linguistic meanings in its sensorimotor behavior. Along this stream, we propose a model that allows the simulated iCub robot to learn the meanings of actions (point, touch, and push) oriented toward objects in robot's peripersonal space. In our experiments, the iCub learns to execute motor actions and comment on them. Architecturally, the model is composed of three neural-network-based modules that are trained in different ways. The first module, a two-layer perceptron, is trained by back-propagation to attend to the target position in the visual scene, given the low-level visual information and the feature-based target information. The second module, having the form of an actor-critic architecture, is the most distinguishing part of our model, and is trained by a continuous version of reinforcement learning to execute actions as sequences, based on a linguistic command. The third module, an echo-state network, is trained to provide the linguistic description of the executed actions. The trained model generalizes well in case of novel action-target combinations with randomized initial arm positions. It can also promptly adapt its behavior if the action/target suddenly changes during motor execution. |
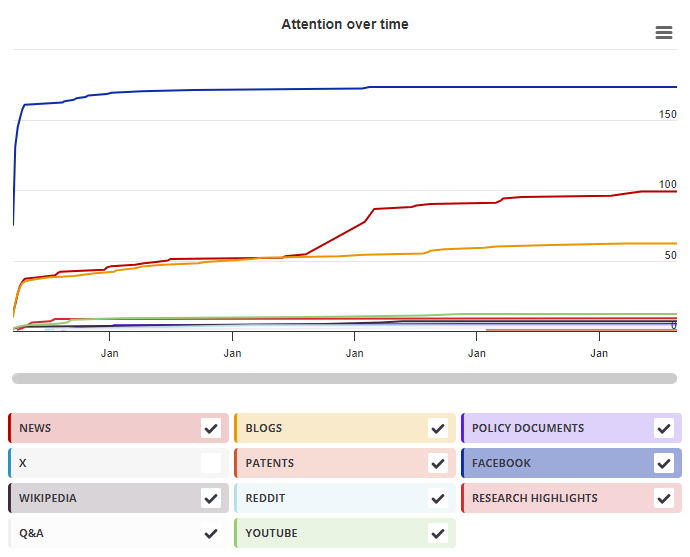
Mendeley readers
Geographical breakdown
Country | Count | As % |
---|---|---|
United Kingdom | 3 | 7% |
France | 2 | 5% |
Germany | 2 | 5% |
Slovakia | 2 | 5% |
Japan | 1 | 2% |
Unknown | 33 | 77% |
Demographic breakdown
Readers by professional status | Count | As % |
---|---|---|
Researcher | 10 | 23% |
Student > Ph. D. Student | 10 | 23% |
Student > Master | 7 | 16% |
Professor > Associate Professor | 4 | 9% |
Student > Doctoral Student | 3 | 7% |
Other | 5 | 12% |
Unknown | 4 | 9% |
Readers by discipline | Count | As % |
---|---|---|
Computer Science | 15 | 35% |
Agricultural and Biological Sciences | 7 | 16% |
Psychology | 6 | 14% |
Engineering | 5 | 12% |
Neuroscience | 3 | 7% |
Other | 2 | 5% |
Unknown | 5 | 12% |