Title |
A generative spike train model with time-structured higher order correlations
|
---|---|
Published in |
Frontiers in Computational Neuroscience, January 2013
|
DOI | 10.3389/fncom.2013.00084 |
Pubmed ID | |
Authors |
James Trousdale, Yu Hu, Eric Shea-Brown, Krešimir Josić |
Abstract |
Emerging technologies are revealing the spiking activity in ever larger neural ensembles. Frequently, this spiking is far from independent, with correlations in the spike times of different cells. Understanding how such correlations impact the dynamics and function of neural ensembles remains an important open problem. Here we describe a new, generative model for correlated spike trains that can exhibit many of the features observed in data. Extending prior work in mathematical finance, this generalized thinning and shift (GTaS) model creates marginally Poisson spike trains with diverse temporal correlation structures. We give several examples which highlight the model's flexibility and utility. For instance, we use it to examine how a neural network responds to highly structured patterns of inputs. We then show that the GTaS model is analytically tractable, and derive cumulant densities of all orders in terms of model parameters. The GTaS framework can therefore be an important tool in the experimental and theoretical exploration of neural dynamics. |
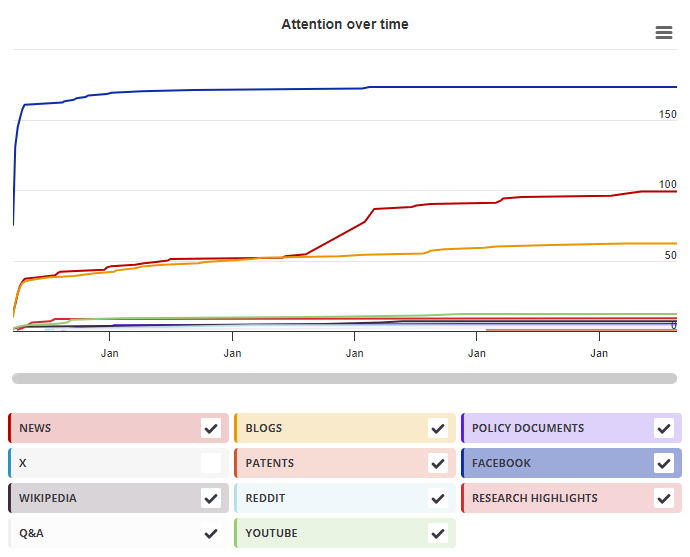
X Demographics
As of 1 July 2024, you may notice a temporary increase in the numbers of X profiles with Unknown location. Click here to learn more.
Geographical breakdown
Country | Count | As % |
---|---|---|
Portugal | 1 | 50% |
Unknown | 1 | 50% |
Demographic breakdown
Type | Count | As % |
---|---|---|
Scientists | 1 | 50% |
Members of the public | 1 | 50% |
Mendeley readers
Geographical breakdown
Country | Count | As % |
---|---|---|
Japan | 1 | 2% |
United Kingdom | 1 | 2% |
Germany | 1 | 2% |
Unknown | 63 | 95% |
Demographic breakdown
Readers by professional status | Count | As % |
---|---|---|
Student > Ph. D. Student | 17 | 26% |
Researcher | 13 | 20% |
Student > Master | 9 | 14% |
Professor | 6 | 9% |
Professor > Associate Professor | 5 | 8% |
Other | 11 | 17% |
Unknown | 5 | 8% |
Readers by discipline | Count | As % |
---|---|---|
Agricultural and Biological Sciences | 16 | 24% |
Physics and Astronomy | 9 | 14% |
Mathematics | 9 | 14% |
Neuroscience | 9 | 14% |
Computer Science | 6 | 9% |
Other | 10 | 15% |
Unknown | 7 | 11% |