Title |
Anisotropic connectivity implements motion-based prediction in a spiking neural network
|
---|---|
Published in |
Frontiers in Computational Neuroscience, January 2013
|
DOI | 10.3389/fncom.2013.00112 |
Pubmed ID | |
Authors |
Bernhard A. Kaplan, Anders Lansner, Guillaume S. Masson, Laurent U. Perrinet |
Abstract |
Predictive coding hypothesizes that the brain explicitly infers upcoming sensory input to establish a coherent representation of the world. Although it is becoming generally accepted, it is not clear on which level spiking neural networks may implement predictive coding and what function their connectivity may have. We present a network model of conductance-based integrate-and-fire neurons inspired by the architecture of retinotopic cortical areas that assumes predictive coding is implemented through network connectivity, namely in the connection delays and in selectiveness for the tuning properties of source and target cells. We show that the applied connection pattern leads to motion-based prediction in an experiment tracking a moving dot. In contrast to our proposed model, a network with random or isotropic connectivity fails to predict the path when the moving dot disappears. Furthermore, we show that a simple linear decoding approach is sufficient to transform neuronal spiking activity into a probabilistic estimate for reading out the target trajectory. |
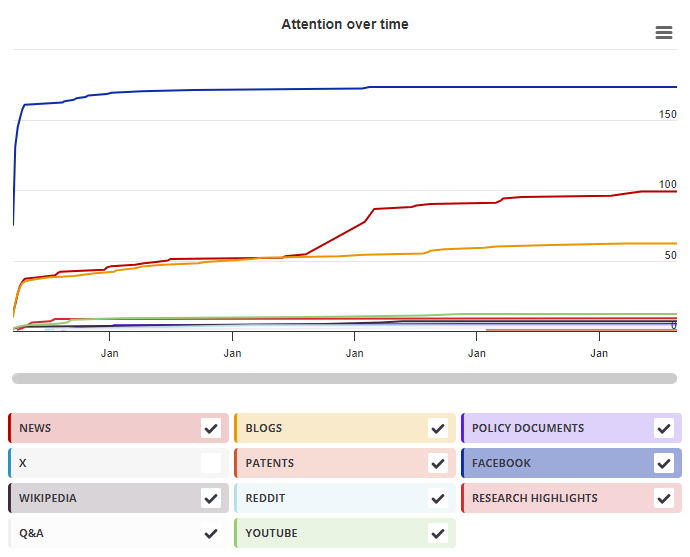
X Demographics
As of 1 July 2024, you may notice a temporary increase in the numbers of X profiles with Unknown location. Click here to learn more.
Geographical breakdown
Country | Count | As % |
---|---|---|
United Kingdom | 1 | 25% |
Unknown | 3 | 75% |
Demographic breakdown
Type | Count | As % |
---|---|---|
Members of the public | 4 | 100% |
Mendeley readers
Geographical breakdown
Country | Count | As % |
---|---|---|
United States | 2 | 3% |
Belgium | 2 | 3% |
Sweden | 1 | 2% |
Germany | 1 | 2% |
France | 1 | 2% |
United Kingdom | 1 | 2% |
Unknown | 50 | 86% |
Demographic breakdown
Readers by professional status | Count | As % |
---|---|---|
Researcher | 18 | 31% |
Student > Ph. D. Student | 12 | 21% |
Student > Master | 9 | 16% |
Student > Bachelor | 4 | 7% |
Professor > Associate Professor | 4 | 7% |
Other | 10 | 17% |
Unknown | 1 | 2% |
Readers by discipline | Count | As % |
---|---|---|
Computer Science | 12 | 21% |
Neuroscience | 11 | 19% |
Agricultural and Biological Sciences | 11 | 19% |
Engineering | 8 | 14% |
Psychology | 6 | 10% |
Other | 7 | 12% |
Unknown | 3 | 5% |