Title |
Nengo: a Python tool for building large-scale functional brain models
|
---|---|
Published in |
Frontiers in Neuroinformatics, January 2014
|
DOI | 10.3389/fninf.2013.00048 |
Pubmed ID | |
Authors |
Trevor Bekolay, James Bergstra, Eric Hunsberger, Travis DeWolf, Terrence C. Stewart, Daniel Rasmussen, Xuan Choo, Aaron Russell Voelker, Chris Eliasmith |
Abstract |
Neuroscience currently lacks a comprehensive theory of how cognitive processes can be implemented in a biological substrate. The Neural Engineering Framework (NEF) proposes one such theory, but has not yet gathered significant empirical support, partly due to the technical challenge of building and simulating large-scale models with the NEF. Nengo is a software tool that can be used to build and simulate large-scale models based on the NEF; currently, it is the primary resource for both teaching how the NEF is used, and for doing research that generates specific NEF models to explain experimental data. Nengo 1.4, which was implemented in Java, was used to create Spaun, the world's largest functional brain model (Eliasmith et al., 2012). Simulating Spaun highlighted limitations in Nengo 1.4's ability to support model construction with simple syntax, to simulate large models quickly, and to collect large amounts of data for subsequent analysis. This paper describes Nengo 2.0, which is implemented in Python and overcomes these limitations. It uses simple and extendable syntax, simulates a benchmark model on the scale of Spaun 50 times faster than Nengo 1.4, and has a flexible mechanism for collecting simulation results. |
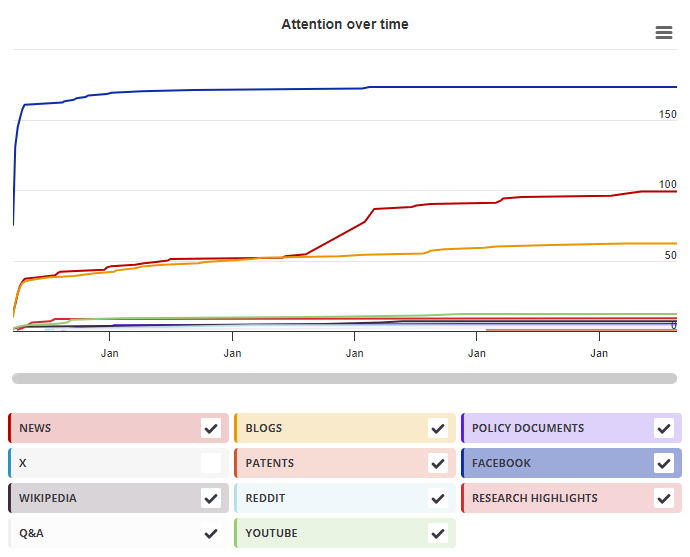
X Demographics
As of 1 July 2024, you may notice a temporary increase in the numbers of X profiles with Unknown location. Click here to learn more.
Geographical breakdown
Country | Count | As % |
---|---|---|
United Kingdom | 3 | 27% |
Canada | 2 | 18% |
United States | 2 | 18% |
Spain | 1 | 9% |
Japan | 1 | 9% |
Unknown | 2 | 18% |
Demographic breakdown
Type | Count | As % |
---|---|---|
Members of the public | 8 | 73% |
Scientists | 2 | 18% |
Practitioners (doctors, other healthcare professionals) | 1 | 9% |
Mendeley readers
Geographical breakdown
Country | Count | As % |
---|---|---|
United Kingdom | 4 | 1% |
Germany | 2 | <1% |
United States | 2 | <1% |
Canada | 2 | <1% |
Australia | 1 | <1% |
South Africa | 1 | <1% |
Turkey | 1 | <1% |
Spain | 1 | <1% |
Belarus | 1 | <1% |
Other | 0 | 0% |
Unknown | 340 | 96% |
Demographic breakdown
Readers by professional status | Count | As % |
---|---|---|
Student > Ph. D. Student | 85 | 24% |
Student > Master | 55 | 15% |
Researcher | 51 | 14% |
Student > Bachelor | 22 | 6% |
Student > Doctoral Student | 15 | 4% |
Other | 45 | 13% |
Unknown | 82 | 23% |
Readers by discipline | Count | As % |
---|---|---|
Computer Science | 79 | 22% |
Engineering | 74 | 21% |
Neuroscience | 43 | 12% |
Agricultural and Biological Sciences | 24 | 7% |
Psychology | 17 | 5% |
Other | 22 | 6% |
Unknown | 96 | 27% |