Title |
Semi-automated Anatomical Labeling and Inter-subject Warping of High-Density Intracranial Recording Electrodes in Electrocorticography
|
---|---|
Published in |
Frontiers in Neuroinformatics, October 2017
|
DOI | 10.3389/fninf.2017.00062 |
Pubmed ID | |
Authors |
Liberty S. Hamilton, David L. Chang, Morgan B. Lee, Edward F. Chang |
Abstract |
In this article, we introduce img_pipe, our open source python package for preprocessing of imaging data for use in intracranial electrocorticography (ECoG) and intracranial stereo-EEG analyses. The process of electrode localization, labeling, and warping for use in ECoG currently varies widely across laboratories, and it is usually performed with custom, lab-specific code. This python package aims to provide a standardized interface for these procedures, as well as code to plot and display results on 3D cortical surface meshes. It gives the user an easy interface to create anatomically labeled electrodes that can also be warped to an atlas brain, starting with only a preoperative T1 MRI scan and a postoperative CT scan. We describe the full capabilities of our imaging pipeline and present a step-by-step protocol for users. |
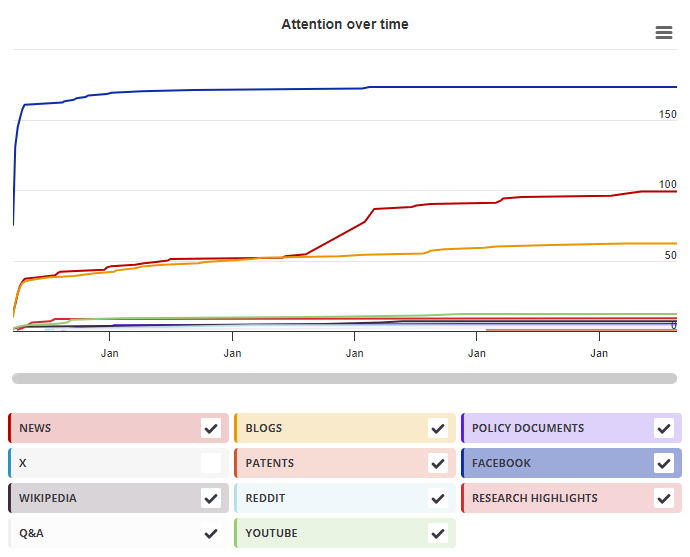
X Demographics
As of 1 July 2024, you may notice a temporary increase in the numbers of X profiles with Unknown location. Click here to learn more.
Geographical breakdown
Country | Count | As % |
---|---|---|
United States | 10 | 24% |
Canada | 2 | 5% |
United Kingdom | 2 | 5% |
Hong Kong | 1 | 2% |
Sri Lanka | 1 | 2% |
Netherlands | 1 | 2% |
Unknown | 25 | 60% |
Demographic breakdown
Type | Count | As % |
---|---|---|
Members of the public | 24 | 57% |
Scientists | 17 | 40% |
Practitioners (doctors, other healthcare professionals) | 1 | 2% |
Mendeley readers
Geographical breakdown
Country | Count | As % |
---|---|---|
Unknown | 92 | 100% |
Demographic breakdown
Readers by professional status | Count | As % |
---|---|---|
Researcher | 29 | 32% |
Student > Ph. D. Student | 12 | 13% |
Student > Master | 11 | 12% |
Student > Doctoral Student | 4 | 4% |
Other | 4 | 4% |
Other | 13 | 14% |
Unknown | 19 | 21% |
Readers by discipline | Count | As % |
---|---|---|
Neuroscience | 28 | 30% |
Medicine and Dentistry | 10 | 11% |
Engineering | 9 | 10% |
Agricultural and Biological Sciences | 7 | 8% |
Computer Science | 5 | 5% |
Other | 9 | 10% |
Unknown | 24 | 26% |